Mitigating Bias in AI
- Reinvented Magazine
- Apr 8, 2023
- 6 min read
Written By; Hana Gabrielle Bidon
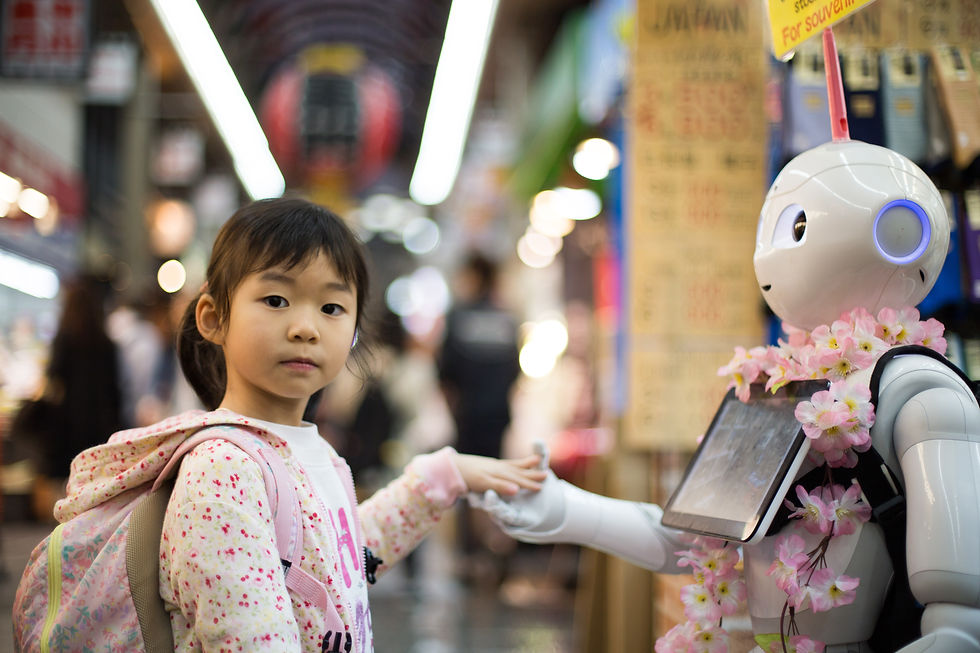
In today’s world, algorithms and their collected data determine a vast range of areas in our lives, ranging from personalized recommendations for movies and TV shows on Netflix to assessing the risk of patients at American hospitals. By automating complex processes, such as hiring qualified candidates for a job, numerous companies, such as Amazon and AT&T, could save time from scheduling interviews with candidates in person. Though algorithmic systems attempt to remove prejudice from the hiring process, these machine learning algorithms “make mistakes and operate with biases” (Diakopoulus, 2015). Algorithmic bias occurs when algorithms generate results built on systematic discrimination due to numerous factors. Examples include the biases of the researchers developing the algorithm(s) and the design process of the algorithm(s).
Biased AI can have serious consequences, as it can perpetuate—and even amplify—existing societal inequalities. When algorithmic systems are trained on biased datasets, they can learn and replicate the same biases and discrimination present in those datasets. An example of biased AI is the development of an AI gaydar, which is an AI system that can supposedly detect someone’s sexual orientation based on their facial features. In February 2017, two Stanford researchers, Yilun Wang and Michal Kosinski, released a research paper called Deep neural networks are more accurate than humans at detecting sexual orientation from facial images, in which they were able to supposedly classify people’s sexual orientation by extracting facial features from more than 35,000 images of straight men, gay men, straight women, and gay women. This facial recognition technology is highly controversial and has received backlash from LGBTQ+ advocacy organizations, such as GLAAD (Gay and Lesbian Alliance Against Defamation) and the Human Rights Campaign, for stigmatizing the LGBTQ+ community. The development of such technology is highly problematic because sexuality is a complex and multifaceted aspect of someone’s identity that cannot be determined based on someone’s appearance. Furthermore, the use of this technology may result in harassment and discrimination towards LGBTQ+ individuals. Therefore, it is essential to be aware of the potential consequences of biased AI and to take action to ensure that algorithmic systems are developed ethically and responsibly.
There are several ways to mitigate bias in AI systems. For example, ensure that the datasets used to train the AI models are inclusive, representative, and holistic. Collecting diverse datasets is crucial in mitigating algorithmic bias in AI systems. Diverse datasets must include a wide range of examples from various sources that represent a variety of perspectives, cultures, and experiences. This ensures that the AI system is trained on an unbiased and broad sample of data instead of a biased and narrow subset of data.
To collect diverse datasets, it is important to consider potential biases within the datasets, including the lack of representation of marginalized groups (e.g., LGBTQ+ people, people with disabilities, etc.), and actively seek ways to address these biases. This may involve partnering with organizations, collecting data from multiple sources, and ensuring that the process of data collection is inclusive and transparent.
Moreover, cleaning and preprocessing datasets are crucial steps to ensure that it is free from inaccuracies and biases. This includes identifying and removing any discriminatory or stereotypical language, images, or information that may be present in the dataset. Attention should also be given to the labels used to categorize the data, as these can influence the AI system's learning and decision-making. For example, using broad and inclusive labels for gender, including gender non-conforming or non-binary, rather than only using gender binary labels like "male" or "female" can help to avoid perpetuating gender stereotypes.
Obermeyer et al. (2019) detected racial bias in a widely used health-risk algorithm and brainstormed three potential reasons for the problem. First, there is a correlation between race and income. People of color—more specifically, Black people—are more likely to have lower incomes compared to White people. In the research paper, Dissecting racial bias in an algorithm used to manage the health of populations, Obermeyer et al. (2019) analyzed around 50,000 patients’ records for a full calendar year, which consisted of “43,539 patients who self-identified as White” and “6079 patients who self-identified as Black.” One of the main problems with this research study is that people who self-identify with other races, such as Middle Eastern, Latino, Asian, and Pacific Islander, were also considered White. Second, poorer patients tend to utilize medical services less frequently or have reduced access to them because of time and location constraints.
Lastly, implicit bias contributes to healthcare disparities. Vartan noted that Black patients receive poorer quality healthcare, which could be attributed to their lack of trust in the healthcare system. Although the advances in machine learning in healthcare streamlined the decision-making process of prioritizing patients with the most complex health needs, there is algorithmic bias embedded in these AI systems, especially against Black people.
One factor in algorithmic bias in healthcare is that there is not enough diverse data to accurately represent the target population. According to a research study from JAMA (the Journal of the American Medical Association), Kaushal et al. (2020), data used to train algorithmic systems in healthcare only came from three states: New York, California, and Massachusetts. One reason for the severe lack of diverse data is that researchers have an arduous time gathering big and diverse medical datasets since they are highly protected by privacy laws. If medical data is erroneously shared, there are severe repercussions and fines. According to a research study from the NCBI (National Center for Biotechnology Information), Tamayo-Sarver et al. (2003), some healthcare providers believe Black patients are less intelligent and have behavioral issues.
Rather than merely pointing fingers at the researchers who unintentionally designed a racially biased algorithm, Obermeyer et al. (2019) improved the risk prediction algorithm to “increase the percentage of Black patients receiving additional help from 17.7% to 46.5%.”
Therefore, collecting diverse datasets is a critical step in mitigating bias in AI systems. By ensuring that the data is inclusive and representative, and by carefully cleaning and processing the data, AI systems can be trained to make more accurate and unbiased decisions.
Another way to mitigate bias is to diversify the field of artificial intelligence, which is mainly led by white men. There needs to be a more inclusive and multidisciplinary culture so that the developed algorithms will reflect the diversity of the population. Though there are affinity groups in artificial intelligence and machine learning, such as Women in Machine Learning and Data Science (WiMLDS), Queer in AI, and Black in AI, peers of AI practitioners (e.g., AI scientists, researchers, and engineers) should learn how to be good allies. Rather than dismissing the experiences of AI practitioners who self-identify as people of color or a gender minority (e.g., women, non-binary femmes), allies should learn when to make space and amplify their work. Additionally, incorporating diversity and inclusion into the teams that develop and deploy AI systems can help to identify and address potential biases. Overall, mitigating bias in AI systems requires a multifaceted approach that involves data collection and curation, ethical guidelines and oversight, and a commitment to diversity and inclusion.
In conclusion, algorithmic bias is a concern in the development and deployment of AI systems. Biased AI can perpetuate existing societal inequalities and lead to unfair and discriminatory outcomes, impacting individuals and communities in numerous ways. However, there are several strategies for mitigating bias in AI systems, including collecting diverse datasets, establishing ethical guidelines, and promoting diversity and inclusion in AI development teams. These strategies can help ensure that AI systems are developed ethically and responsibly, and that they are fair, transparent, and inclusive. It is crucial that we address algorithmic bias to ensure that AI technology is used in a way that benefits all members of society regardless of their backgrounds.
References
Obermeyer, Z., Powers, B., Vogeli, C., & Mullainathan, S. (2019). Dissecting racial bias in an algorithm used to manage the health of populations. Science, 366(6464), 447–453. https://doi.org/10.1126/science.aax2342
Kaushal, A., Altman, R., & Langlotz, C. (2020). Geographic distribution of us cohorts used to train deep learning algorithms. JAMA, 324(12), 1212. https://doi.org/10.1001/jama.2020.12067
Whittaker, M., Alper, M., Bennett, C., Hendren, S., Kaziunas, L., Mills, M., Ringel Morris, M., Rankin, J., Rogers, E., Salas, M., & Myers West, S. (s. d.). Bias at the Intersection of AI and Disability. In Disability, Bias, and AI. AI Now Institute at NYU. Consulted 26 Feb 2023, at the address https://ainowinstitute.org/disabilitybiasai-2019.pdf (Original work published 2019)